Large Language Models Won’t Save Army’s Data Overload
Data is critical for a connected Army, but managing datasets will require technologies that are useful at the tactical edge.
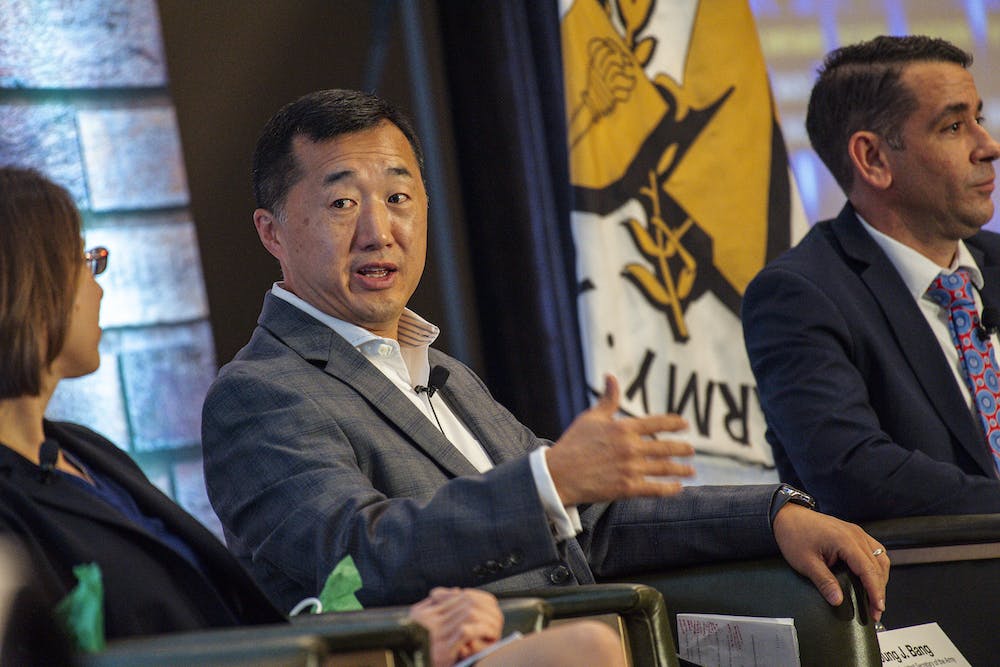
Data is critical for a global connected U.S. Army, but as the service ingests data from disparate sources and battlefields all over the world, Army leaders want to ensure it is not inundated with data that it cannot use.
“There’s too much damn data out there, and we can’t overload our warfighters and our leaders with too much data,” Army Principal Deputy Assistant Secretary for Acquisition, Logistics and Technology Young Bang said at an AUSA Cyber & Information Advantage Hot Topic event earlier this month.
Michael Diorio, senior vice president of global operations at Dataminer, added that data can be collected from sources as diverse as sensors, tweets, videos, photos, audio broadcasts or transponders. As billions of data points are ingested to one location, the totality of information can give a clear picture of a location or event.
“It’s been talked about for years that data is the oil, but at the same time, there wasn’t necessarily the technology to really analyze all this data, look at causal inference and then correlations. And so what we do with our large language models and foundation models is really look at the strength of signal that is happening around an event,” Diorio said.
Edward Kao, research scientist at MIT Lincoln Laboratory, said to generate an information advantage for the Army, the public and private sectors will need to use the potential of generative AI.
“Our adversaries will be using and are already using generative AI. I think there’s ethical issues about using generative AI to put out content, but in terms of using generative AI at least to automate, the understanding of information landscape is critical. I don’t think we really have a choice there,” Kao said.
He added that generative AI is unlikely to be usable in the short term as a fully automated tool, and that the interaction between humans and AI in using it will be absolutely “critical.”
“I think what a human analyst offers is far more than just giving approval to the content. I think a human analyst actually provides the coloring and the context and the mission interpretation that I just don’t think we can expect a machine to be able to do that,” Kao said. “I think the machine is really good at aggregating information, so when the human applies that interpretation, you’re doing it at a mass scale. It’s to scale up that interpretation, but not actually making that interpretation.”
Stephen Riley, of the Army engineering team at Google, said that despite data overload, large language models (LLM) will rarely be effective for the Army.
“Ninety percent of the time, don’t do it. It’s the easy button, I know, but using LLMs, that is boiling the ocean to make yourself a cup of coffee. You don’t have the compute resources to run effective LLMs down at the tactical edge,” Riley said.
Riley encouraged leaders to look toward the “old ways of doing things” like knowledge graphs as a more effective way to manage and aggregate data, rather than jumping for complex technologies that require more compute power.
“You could actually encode all of those [automatic data processes], all the operations stuff, all the intel stuff. We could encode that into a knowledge graph, which requires, I’ll just be hyperbolic, infinitely less compute power. That’s something you could deploy forward on a pretty small box.”
Riley added that LLM hallucination is also a real issue, but warned that just because humans oversee an LLM, that does not automatically make it a valid dataset.
“Google is biased. Everybody’s biased. You’ve got to look out for that too,” Riley said. “Who is the one that’s the gatekeeper of the shifting of the Overton Window? Whose values are you implicitly encoding in the data set that you’re now using?”
Riley warned that as government acquires AI technology and datasets gathered from the commercial world, it is incumbent on the government to “demand to see where the data came from.”
“We have already seen cases where companies building large LLMs have sourced data from other companies that say they have a bunch of data. And it turns out they source from other companies that are given some pretty bad stuff. Maybe not deliberate misinformation, but stuff that absolutely would not comply with our nation or Army values,” Riley said.
This is a carousel with manually rotating slides. Use Next and Previous buttons to navigate or jump to a slide with the slide dots
-
Federal Leaders Revamp Tech Workforce, Policy
Despite the rise in interest of emerging technology, federal leaders see data, policy and the workforce as a best vehicle for change.
4m read -
Looking Back at the First Trump Administration's Tech Priorities
In his first term, Donald Trump supported cybersecurity, space policy and artificial intelligence development.
4m read -
Labor CAIO Outlines Responsible and Ethical AI Priorities, Use Cases
Department of Labor Chief AI Officer Mangala Kuppa outlined how her role is shaping the agency’s artificial intelligence strategy.
20m watch -
Elevating Cybersecurity in the Intelligence Community
The Intelligence Community is developing strategies to protect data and strengthen resiliency against emerging cyber threats.
30m watch