Navy Needs Machine Learning to ‘Operate at the Wet Edge’
The service requires connectivity, data and machine learning to modernize its expeditionary force.
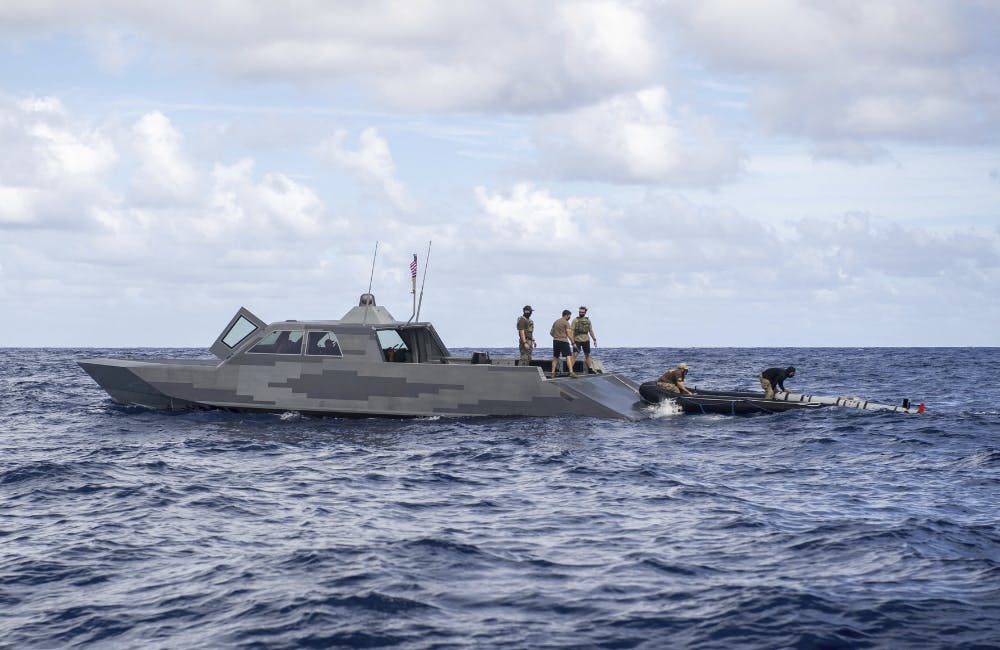
For the U.S. Navy, operating at the wet edge requires communications and connectivity, accessible and usable data, robust automated target recognition systems and critical partnerships to overcome challenges associated with deploying capabilities in harsh, unpredictable and sometimes dangerous conditions.
While operating both at the edge and at the wet edge is highly challenging due to extreme environments, the treacherous and precarious nature of the ocean environment increases the risk of various types of accidents.
“Operating at the wet edge is worth drawing out some distinctions for a couple of reasons. It’s chaotic. It’s uncertain. It is challenging for sensors to gather high-fidelity information underwater,” Capt. Jon Haase, program manager of the NAVSEA Expeditionary Missions program office (PMS 409), said at the NDIA’s 25th Annual Expeditionary Warfare Conference last week. “It is a wild environment with limited communication. And so, for that reason, it’s the perfect environment to operate our machine learning algorithms, our autonomy and our fleet of underwater robotic systems.”
PMS 409 has adopted several pillars crucial to operating at the wet edge environment with autonomy, including advanced technologies and a well-trained workforce.
Connected systems
The Expeditionary Missions program office completed the MK 18 Mod 2 Unmanned Underwater Vehicle (UUV) program earlier this month. The program started production over a decade ago and has delivered over 90 MK 18 Mod 2 UUV vehicles to the fleet since the initial launch.
These UUVs allow the Navy to perform a wide range of missions in an underwater environment, such as reconnaissance, search and rescue. Using various sensors, UUVs gather data about the underwater environment that is valuable for future operations.
It is crucial to the program’s success to have connected systems to operate reliably and fulfill its missions.
“[UUV] is tasked with searching large swaths underwater and locating objects of interest, that’s not possible for one vehicle to do. These vehicles must communicate with other vehicles at the same time. But we operate a family of systems so these vehicles must communicate with vehicles of different types,” Haase said. “Taking that one step further, vehicles must be able to communicate with robotic systems that do other tasks as well in order for us to complete a kill chain. That connection and connectedness of these systems turns out to be critical for our program in the efforts.”
Data-efficient learning
In naval operations where fast and accurate decision-making is crucial, machine learning helps Haase’s team operate at the wet edge by improving the speed of decision-making in challenging environments.
“Ideally, we could look at things like a zero-shot, one-shot, limited amounts of data — that is not the world we live in at the moment. Data efficient representation learning is critical for us,” Haase said.
It requires accessible, clean and labeled data to perform data efficiency learning. “Once this data is stored, labeled and accessible, our developers can work with it to generate the sort of automated target recognition necessary,” Haase said.
Robust automatic target recognition
Haase’s team requires automatic target recognition systems that function effectively in any environment. This technology provides the Navy with such capabilities as target identification and classification.
“What we think that means is we’ll need a fleet of models that work and are well generalized,” Haase said. “In order to do that, we will need model registries and a number of best practices from industry that will allow us to track which models to use in which environments – an ongoing challenge for scaling machine learning solutions.”
Reduced data deployment timeline
To operate at the wet edge, Haase emphasized the need to reduce the time it takes to receive and deploy data to help make decisions rapidly.
“The time from data to deployment needs to be compressed so that in operational environments, when we see critical new datasets and data points for the first time, we can take ingest those into a machine learning pipeline and rapidly turn that into a solution that we can put back in those robotic systems,” Haase said.
Partnerships
The Expeditionary Missions program office has partnered with the Defense Innovation Unit on a number of projects that provided Haase’s team with the ability to bring data center tools and machine learning environmental improvements to scale in the Defense Department.
“We found that their technical expertise, their innovation, their connections to industry, and their flexible contracting approach was critical for us to gain agility in this effort,” Haase said.
This is a carousel with manually rotating slides. Use Next and Previous buttons to navigate or jump to a slide with the slide dots
-
HHS Aligns AI, Tech Strategy Under its Policy Agency
ONC will have a new name and oversee more c-suites to better shape the future of health care technology policy.
3m read -
Defense Board Calls for Incentives to Faster Tech Adoption, Innovation
The group supports recommendations that would support innovators at the department and optimize collaboration.
4m read -
The Future of AI at the Defense Department
To maintain its competitive edge, the Defense Department is exploring artificial intelligence across the enterprise.
14m read -
Large Language Models Won't Save Army's Data Overload
Data is critical for a connected Army, but managing datasets will require technologies that are useful at the tactical edge.
6m read