NIH Makes Progress in AI Efforts for Research
A working group’s recommendations for artificial intelligence can propel biomedical research.
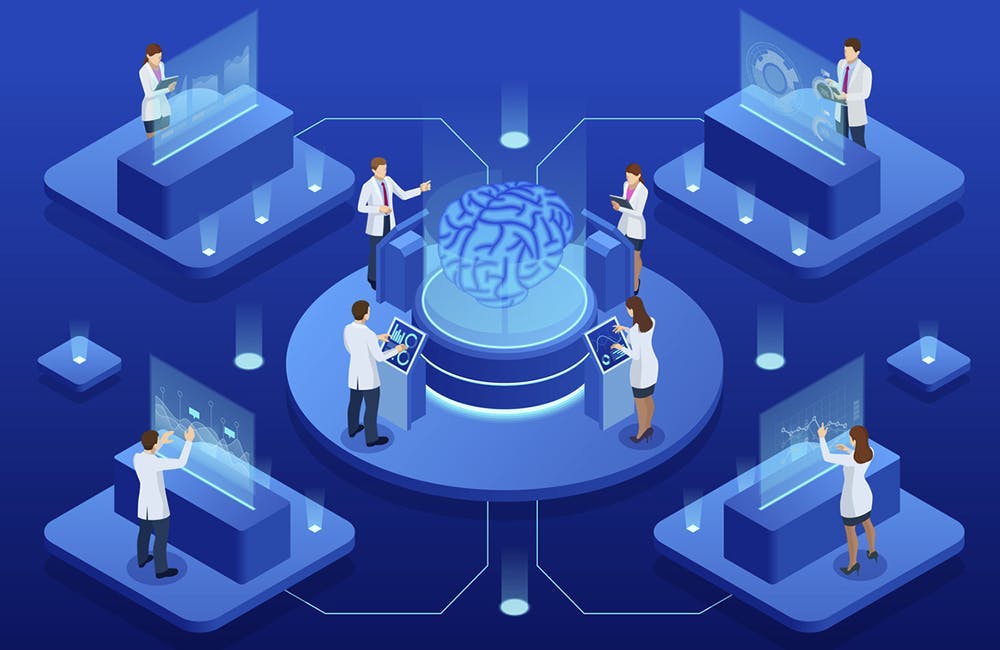
A working group tasked with addressing best practices and guidelines for the National Institutes of Health to advance artificial intelligence in research efforts released recommendations last month to address areas like data generation and ethics around the technology.
Artificial intelligence provides many opportunities to assist in biomedicine, such as interpreting unstructured text and data in medical records and detecting cancerous tumors with increased speed and accuracy. Without proper guidance, it could produce less than optimal results or lag behind its development.
To maximize the potential of the emerging technology, NIH created the AI Working Group in December 2018, comprising government, industry and academic research experts. A year later, the group delivered eight recommendations at the Advisory Committee to the Director meeting at the NIH Campus in Bethesda, Maryland.
The group’s recommendations to propel progress in AI for biomedical science and research are:
- Support flagship data generation efforts to propel progress by the scientific community.
- Develop and publish criteria for machine learning-friendly datasets.
- Design and apply “datasheets” and “model cars” for biomedical machine learning.
- Develop and publish consent and data access standards for biomedical machine learning.
- Publish ethical principles for the use of machine learning in biomedicine.
- Develop curricula to attract and train machine learning-biomedicine experts.
- Expand the pilot for machine learning-focused trainees and fellows.
- Convene cross-disciplinary collaborators.
Group Co-Chair David Glazier, director of Verily Life Sciences, Alphabet Inc.’s research organization and formerly part of Google X, presented the group’s final AI recommendations to NIH Director Francis Collins and his committee.
“If you could sort of pick an area that has emerged in the last half-decade or so that is going to have a really massive impact on biomedical research that we need to pay close attention to, this is probably it,” Glazier emphasized during his presentation introduction.
He noted two revolutions shaping how the world thinks about technology: generating data and analyzing it. This encompasses biomedical data generated specifically for machine learning and also machine learning that is designed for biomedical experiments, which need harmonization of data, people and ethics.
Many datasets lack crucial metadata, such as information collected on research participant demographics due to poor experimental design, Glazier explained, but the data collection methods themselves are restrictive and require reworking to support machine learning.
A lack of representation in research and small sample sizes can also lead to unethical and ill-formed medical conclusions with large implications in health care applications, such as in developing treatments. As there are existing inequities in medicine, Glazier said, these could be especially amplified with the involvement of artificial intelligence algorithms that may incorrectly explain or perpetuate those problems.
“How should [research data collection] be done in a way that maximizes the value to all populations by making sure the data on all populations is part of building these new insights and interventions, while at the same time protecting all populations?” he said. “That’s a challenge to get that right, to get that balance right.”
Aside from challenges in using artificial intelligence on existing data, the health agency also discussed ways to train, hire and retain people who work in biomedicine and computer science.
Comparing effective collaboration between groups of professions to “herding cats,” Glazier said that “none of our systems train people to be able to work across these disciplines.” He added that computational scientists often aren’t aware of the most useful problems to solve, while biomedical scientists aren’t aware of what tools are available or useful for their research problems. Paired with disjointed educational systems, it is increasingly difficult to get the right people together and create opportunities for cross-pollination of ideas and solutions.
Concerns about people were further echoed by the committee.
For one, quality graduate students and AI experts will be difficult to attract if they are unaware of the unique opportunities NIH can provide them, said Dina Katabi, a computer science professor at Massachusetts Institute of Technology.
High-paying salaries are frequently a secondary concern for many people applying to NIH, but the primary concern is whether they can generate high-impact work within the agency, Katabi suggested. For instance, potential employees may opt to work at places besides NIH if there’s a perception that the agency’s projects will be less impactful or fulfilling than those they could work on elsewhere.
In response to these concerns, Glazier emphasized the importance of raising awareness around the agency’s overarching mission as well as its massive collection of datasets and tools to attract prospective candidates. Additionally, cultivating existing people and successfully collaborating across disciplines should also be top priorities for the agency.
Though the discussion within the committee was largely workforce-driven, the multifaceted recommendations were unanimously approved by the ACD.
Following its decision, NIH will begin the process of transforming the recommendations into measurable actions, Collins said after the committee vote. The AI Working Group will continue to meet informally for further agency guidance and support, as needed, he said.
This is a carousel with manually rotating slides. Use Next and Previous buttons to navigate or jump to a slide with the slide dots
-
The CAIOs Leading Responsible AI Development Across Government
Since the White House's AI executive order, federal agencies are in the process of naming chief artificial intelligence officers.
7m read -
Defense Board to Pitch Solutions for Closing Tech Talent Gaps
Defense Innovation Board members cite need to modernize people management the same way government modernizes technology.
4m read -
Energy Researchers Aim For Holistic Approach to AI Issues
A new center at the Oak Ridge National Laboratory is looking at under-researched areas of AI to better understand how to secure it.
2m read -
5 Predictions for AI in Government Technology
Agencies are setting plans in motion not only to integrate AI into their enterprises, but also ensuring the data that power these systems are fair.
41m watch