Effective AI Requires Constant Monitoring, AI Leaders Say
Refining and reviewing data models is essential for impactful application of artificial intelligence.
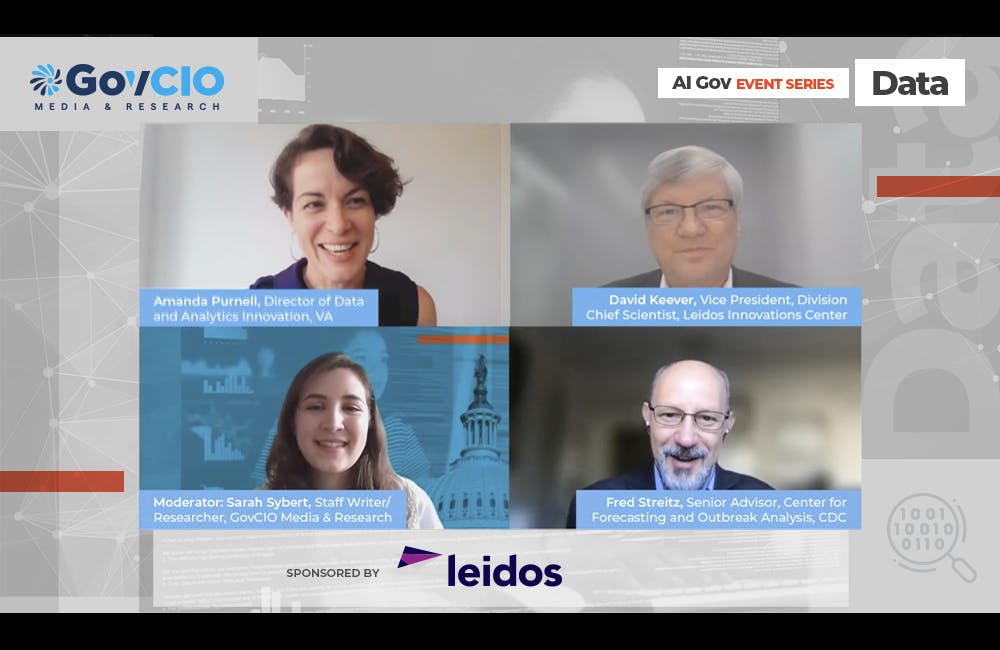
Federal data leaders expounded on their testing strategies for artificial intelligence (AI) models to ensure accuracy and relevancy at GovCIO Media & Research’s AI Gov: Data event Thursday.
AI models are generally only as effective as the data used to uphold them. In cases where data is not continually refreshed, or its limitations unaddressed, conclusions may be inaccurate. Sometimes even if the data is accurate and current, AI models can fail to account for discrepancies or variations in data and produce flawed results.
The Department of Veterans Affairs has made recent investments in AI and uses advanced modeling for public health and biomedical research. Data leadership overseeing these initiatives have become increasingly attuned to the need to review their models for biases, and noted the potential limitations of a particular data set to ensure the results are interpreted and applied correctly.
“You really do need to test models – systematically, strategically, over and over again, with different sub populations and even with different VA hospitals,” said Amanda Purnell, VA Director of Data and Analytics Innovation, at the event. “So there is no one model for the VA. That wouldn’t work. Because people are different by region, different by area, different by health condition. So we have to continuously test and retest models. And we have the computer power to do this incredible work with reconfiguring, readjusting, developing specialized and tailored models for different groups of people.”
Other health and science-focused agencies have worked to apply similar review standards to their AI projects, including the Centers for Disease Control and Prevention’s (CDC) efforts to model the spread of COVID-19. This requires large-scale recalibration when new variants emerge to ensure projections account for the virulence and pathology of new strains.
“There is no single model that is applied. We apply a model which is usually trained on a specific set of data to address a specific question,” said Fred Streitz, Senior Advisor at the CDC Center for Forecasting and Outbreak Analysis, at Thursday’s event. “And those models are recalculated constantly, and the turnaround on that is hours or days. So as new variants emerge, we respond to that very quickly and retrain models as necessary to address specific questions. But there is no there is no one model to rule them all. There is no one forecast that’s absolutely correct. Everything is in the context in which it was built and the information that went into the model.”
The unifying process behind all these initiatives is ongoing review of data inputs, which helps ensure the integrity of AI models’ conclusions.
“This is an iterative process and models get built and used by individuals, but there’s this continuous monitoring of the models that also needs to occur,” said David Keever, Vice President and Division Chief Scientist at the Leidos Innovations Center, at Thursday’s event.
This is a carousel with manually rotating slides. Use Next and Previous buttons to navigate or jump to a slide with the slide dots
-
Navigating Zero Trust for Cybersecurity
The World Bank Group and Lumen advance zero-trust strategies and identity management to secure sensitive data.
18m watch -
DIU Platform to Link Private Sector Innovation with Defense Needs
Digital OnRamp consolidates databases and uses AI to simplify the discovery process for both companies and DOD users.
5m read -
The CAIOs Leading Responsible AI Development Across Government
New AI memos from the Trump administration prompt federal agencies to establish chief AI officers and OMB to launch a new CAIO AI Council.
7m read -
How AI and Data Are Shaping the Future of the Coast Guard
Deputy CIO Brian Campo says USCG is focusing its AI on efficiency, learning from peers and simplifying regulations for the maritime community.
17m watch