No Blinking: How AI Could Better Handle Large-Scale Surveillance
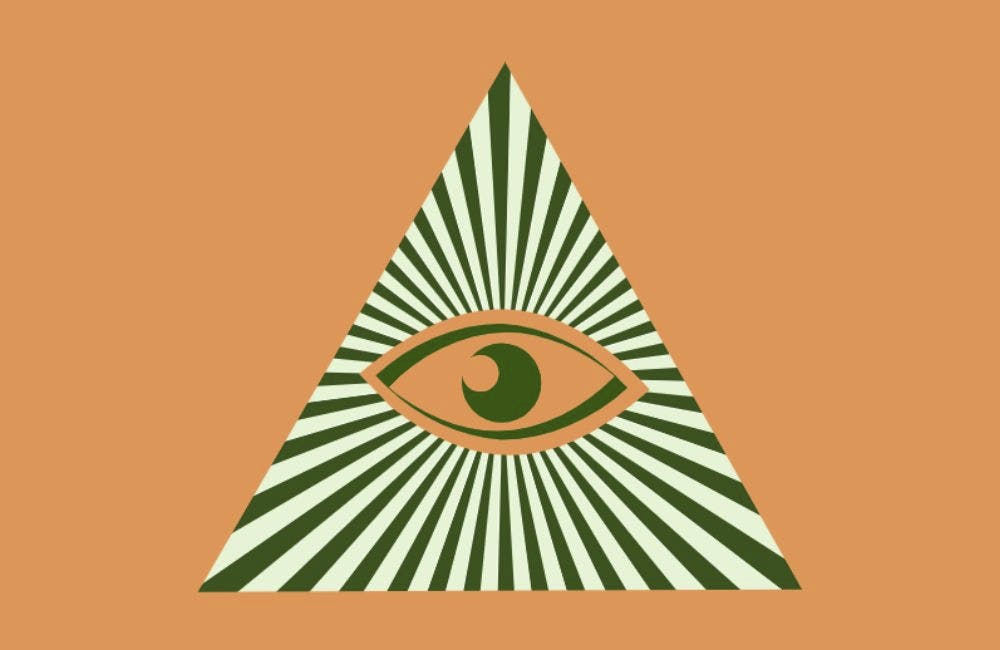
Pity the poor security guard parked behind a desk framed by eight or 10 CCTV feeds of parking lots, hallways and doorways, spending every night binge-watching the dullest reality TV show imaginable. Once in a while, something happens, and the perpetual feeds from those cameras can alert the guard of nefarious activity and initiate a response. But if the guard happens to be looking the other way or getting some coffee at the wrong time, someone or something just might slip by. Despite hours of watching monitors, an opportunity is missed.
Multiply the number of cameras by 10, 100, or a few thousand, and you have an idea of what the government is looking at when it comes to getting the best possible results from its video surveillance. Whether it’s reviewing terabytes of video data collected or trying to keep track of what’s happening in real time, agencies don’t have enough eyeballs to take a good look at everything its cameras see.
— Sign up for our weekly newsletter to receive the latest analysis and insights on emerging federal technologies and IT modernization.
So, it is turning to machines to do the heavy looking for them, both in culling through endless hours of accumulated full-motion video or keeping a real-time eye on surveillance feeds from secure government facilities, transportation hubs and other areas. Artificial intelligence, machine learning and neural networks hold a lot of promise for this kind of work — they don’t blink, get coffee or have to go to the restroom — though progress is still in the early stages.
Learning to Watch TV
The Defense Department’s Project Maven, for instance, is looking to tap into artificial intelligence technology — specifically, computer vision algorithms, machine learning and big data analytics — to automate analysis of the millions of hours of video collected by drones in Iraq and Syria. One early outcome of the project is data-tagging technology that trains machines to identify certain objects — a color of hat, a type of vehicle, a person carrying a weapon — in still images.
But Project Maven and other efforts like it (in both the public and private sectors) are still trying to perform forensic analysis — sorting through collected images and videos. A project run by the Intelligence Advanced Research Projects Activity is looking to go one step further. The Deep Intermodal Video Analytics, or DIVA, project aims to improve the speed and accuracy of analyzing collected video, but also wants to be able to produce real-time alerts when the system recognizes pre-defined activities that could be a sign of threats.
“There is an increasing number of cases where officials, and the communities they represent, are tasked with viewing large stores of video footage, in an effort to locate perpetrators of attacks, or other threats to public safety,” Terry Adams, DIVA program manager, said in announcement. “The resulting technology will provide the ability to detect potential threats while reducing the need for manual video monitoring.” (Aware of the popular perception of intelligence community projects, Adams also added the disclaimer that DIVA’s technology will not track individuals’ identity and will be designed to protect personal privacy.)
What to Watch For
DIVA focuses on what is calls primitive activities — a person getting into or out of a vehicle, or carrying an object — as well as more complex activities, such as someone being picked up by a vehicle, abandoning a package or other object, two people exchanging an object, or someone carrying a firearm.
The project’s first phase will deal with video collected in light visible to the human eye from indoor and outdoor cameras with either a fixed range or with limited pan-tilt-zoom motion. Phases two and three will expand into video collected from handheld or wearable cameras and include a broader spectrum of light, including infrared.
In its original solicitation, IARPA said it expected collaborative, multidisciplinary teams from industry and academia with skills ranging from machine learning, deep learning and AI, to detection of people and objects, and 3-D reconstruction from video, superresolution, statistics, probability and mathematics. IARPA isn’t looking for a stand-alone or proprietary system, but a scalable framework used in an open cloud environment, incorporating an array of cameras whose fields of vision may or may not overlap.
IARPA has picked six teams to work on new research for DIVA. Kitware Inc. and the National Institute of Standards and Technology will test the new systems.
This is a carousel with manually rotating slides. Use Next and Previous buttons to navigate or jump to a slide with the slide dots
-
DOD Has a New Cyber Resiliency Assessment Program
Defense officials tout the continuous assessment feature and scalability of the new program amid increased cyber threats.
5m read -
Transitioning Systems for Modern Agency Missions
IT modernization is a constant process necessary for improving customer service, mission delivery and collaboration.
40m watch -
Cyber Resilience and Recovery Amid Evolving Cyber Threats
Data durability is a key aspect of NIST’s cybersecurity framework for public and private organizations.
21m listen -
How Tech Enables Environmental Justice at EPA
The agency wants to eliminate bias and establish new tech standards to reduce greenhouse gas emissions.
39m listen